Challenges in Implementing Predictive Analytics
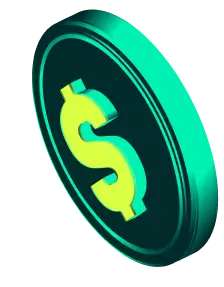
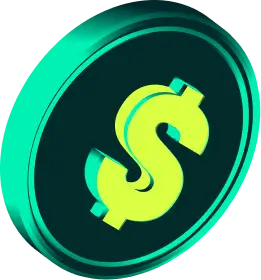
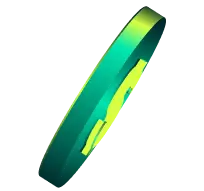
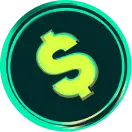
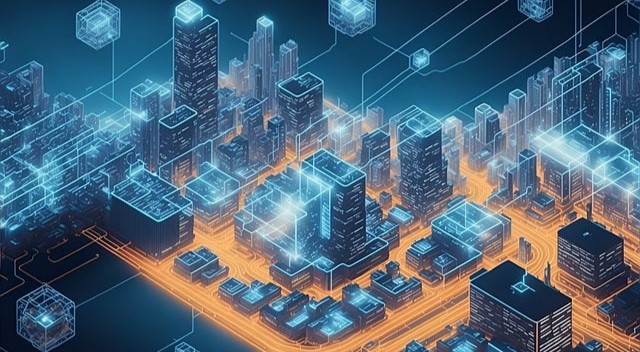
Challenges in Implementing Predictive Analytics
Predictive analytics plays a pivotal role in modern personalization strategies by using data, statistical algorithms, and machine learning techniques to anticipate customer needs and behaviors. By analyzing historical data and identifying patterns, businesses can proactively deliver tailored experiences that resonate with individual customers, enhancing engagement, satisfaction, and conversions. Here’s a detailed exploration of its role:
1. Understanding Predictive Analytics in Personalization
Predictive analytics helps businesses:
- Anticipate customer needs before they explicitly express them.
- Optimize timing and delivery of offers or content to maximize impact.
- Refine personalization strategies through continuous learning and adaptation.
Examples include recommending products, predicting churn risk, or tailoring communication based on likely customer preferences.
2. Data Sources Used in Predictive Analytics
To build effective predictive models, businesses leverage various data types:
- Demographic Data: Age, gender, income, location.
- Behavioral Data: Browsing history, clickstreams, purchase patterns, engagement with past campaigns.
- Transactional Data: Purchase frequency, average order value, preferred payment methods.
- Sentiment Data: Social media activity, reviews, and customer feedback.
- Contextual Data: Device usage, time of day, geolocation, and weather conditions.
3. Techniques in Predictive Analytics for Personalization
Machine Learning Algorithms:
- Use supervised and unsupervised learning to identify customer segments and predict future actions.
- For example, clustering algorithms can group customers with similar preferences, enabling targeted recommendations.
Predictive Models:
- Recommendation Systems: Suggest products, services, or content based on historical interactions and similar customer profiles.
- Churn Prediction Models: Identify customers at risk of leaving and trigger personalized retention campaigns.
- Lifetime Value Prediction: Forecast a customer’s potential value to prioritize high-impact personalization efforts.
Natural Language Processing (NLP):
- Analyze text-based data, such as reviews or social media comments, to understand customer sentiments and preferences.
4. Applications of Predictive Analytics in Personalization
1. Personalized Product Recommendations:
- Suggest items based on past purchases, browsing history, and similar customer behaviors.
- Example: Amazon’s “Customers who bought this also bought” feature.
2. Anticipating Customer Needs:
- Predict when a customer may need to reorder consumables (e.g., groceries or medicine) and send reminders or discounts.
- Example: Subscription services proactively suggesting refills before customers run out.
3. Optimizing Marketing Campaigns:
- Personalize email, SMS, or ad campaigns by predicting which offers or content segments will resonate most with specific users.
- Example: Tailoring holiday promotions to customers who typically shop during sales events.
4. Dynamic Pricing:
- Predict customer price sensitivity and adjust pricing strategies accordingly to maximize sales or margins.
- Example: Airlines offering personalized ticket prices based on demand patterns.
5. Enhancing Customer Support:
- Predict the type of assistance a customer might need based on their interaction history and automate support suggestions or chatbot responses.
- Example: A telecom company anticipating billing-related queries and offering proactive resolutions.
6. Retargeting Campaigns:
- Predict which users are most likely to convert after abandoning a cart or browsing a product and target them with personalized offers.
- Example: Sending a discount code to customers who recently viewed high-ticket items but didn’t purchase.
7. Cross-Selling and Upselling:
- Recommend complementary or premium products based on a customer’s past purchases and predicted interests.
- Example: Suggesting accessories for electronics or premium subscriptions for digital services.
5. Benefits of Predictive Analytics in Personalization
- Enhanced Customer Experience: Anticipating needs makes interactions feel intuitive and effortless.
- Increased Engagement: Relevant recommendations and timely interventions capture customer interest.
- Higher Conversion Rates: Predicting and acting on customer intent drives more purchases or desired actions.
- Improved Retention: Identifying at-risk customers and addressing their concerns enhances loyalty.
- Cost Efficiency: Focused efforts on high-value customers or prospects reduce marketing waste.
6. Challenges in Implementing Predictive Analytics
- Data Quality and Integration: Predictive accuracy relies on comprehensive, clean, and well-integrated data.
- Privacy Concerns: Businesses must balance personalization with respect for customer privacy and comply with regulations like GDPR and CCPA.
- Model Accuracy: Continuous monitoring and updating of models are required to account for changing customer behaviors and preferences.
- Scalability: Handling large datasets and implementing real-time predictions across multiple channels can be resource-intensive.
7. Tools and Technologies for Predictive Personalization
- AI Platforms: Tools like Salesforce Einstein, Google Cloud AI, and AWS Machine Learning for building predictive models.
- Recommendation Engines: Platforms like Dynamic Yield, Algolia, or Adobe Target to deliver real-time recommendations.
- Data Visualization: Tools like Tableau or Power BI for interpreting predictive analytics outputs and driving decisions.
8. Best Practices for Success
- Start Small: Begin with specific use cases (e.g., product recommendations) and expand as models prove effective.
- Test Continuously: A/B test predictive outputs to ensure accuracy and relevance.
- Focus on Value: Use predictive analytics to enhance value for customers, not just drive sales.
- Communicate Transparency: Explain how personalization improves the customer experience to build trust.
By harnessing predictive analytics, businesses can anticipate customer needs, create hyper-personalized experiences, and stay ahead of competitors in delivering value. This approach transforms customer interactions from reactive to proactive, resulting in deeper relationships and increased business performance.