Predictive Analytics in Marketing: Forecasting Customer Behavior and Enhancing Marketing Strategies
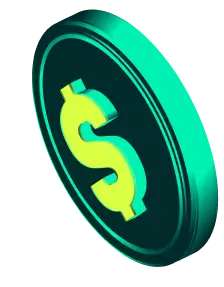
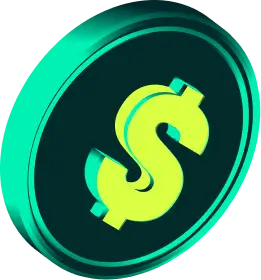
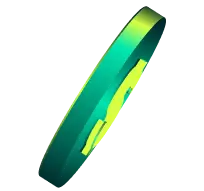
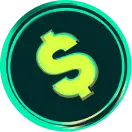
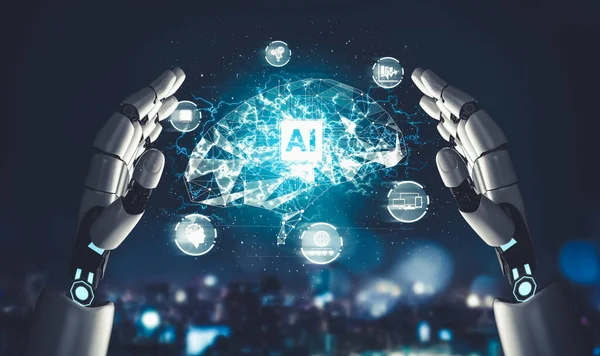
Predictive Analytics in Marketing: Forecasting Customer Behavior and Enhancing Marketing Strategies
Predictive Analytics in Marketing: Forecasting Customer Behavior and Enhancing Marketing Strategies
Predictive analytics is a powerful tool in the marketing landscape, enabling businesses to forecast customer behavior and make data-driven decisions that enhance marketing strategies. By leveraging historical data, statistical algorithms, and machine learning techniques, predictive analytics can help businesses anticipate what customers are likely to do next. This allows for more personalized, timely, and effective marketing efforts.
1. What is Predictive Analytics in Marketing?
Predictive analytics involves using historical data, statistical modeling, and machine learning algorithms to predict future events or behaviors. In marketing, it is used to forecast customer actions, such as purchasing decisions, churn likelihood, or engagement patterns. These insights help marketers develop proactive strategies, enhance customer experiences, and optimize marketing spend.
2. How Predictive Analytics Enhances Marketing Strategies
By leveraging predictive analytics, marketers can make informed decisions that improve various aspects of their strategies. Here’s how predictive analytics can directly enhance marketing efforts:
a. Customer Segmentation and Targeting
Predictive analytics allows businesses to segment their customers based on predicted behaviors, such as the likelihood of making a purchase, the expected lifetime value, or the risk of churn. By analyzing historical data, marketers can identify distinct customer segments with similar characteristics or needs and target them with tailored marketing campaigns.
For example:
- High-value customers: Marketers can target customers predicted to generate significant revenue with exclusive offers or loyalty rewards.
- At-risk customers: Those predicted to churn can be identified early, allowing marketers to send retention-focused emails or personalized discounts to re-engage them.
b. Personalized Marketing Campaigns
Predictive analytics enhances the ability to deliver personalized messages to customers. By predicting customer preferences, behaviors, and purchasing patterns, marketers can create tailored content, product recommendations, and offers that resonate with each individual.
For instance:
- A clothing retailer can predict which styles a customer is most likely to buy based on previous purchases and browsing behavior, offering targeted ads or email campaigns with relevant product suggestions.
- A travel company might predict destinations that appeal to a customer based on past bookings and send personalized travel deals or promotions.
c. Lead Scoring and Conversion Optimization
Predictive analytics can improve lead scoring by identifying which leads are most likely to convert into paying customers. By analyzing past interactions and behaviors (such as website visits, email opens, and content downloads), businesses can score leads based on their likelihood to convert. This allows sales and marketing teams to prioritize high-potential leads, focus efforts on the most promising prospects, and increase the efficiency of conversion strategies.
For example:
- A SaaS company might use predictive analytics to determine which trial users are likely to become paying subscribers based on their activity and engagement with the platform. This allows the sales team to focus on high-probability leads.
d. Optimizing Marketing Campaigns and Budget Allocation
Predictive analytics can forecast the performance of different marketing campaigns and channels, helping businesses allocate their marketing budgets more effectively. By analyzing data from past campaigns, marketers can predict which strategies will yield the best ROI, identify underperforming tactics, and shift resources accordingly.
For instance:
- Predictive models can determine the most effective channels (email, social media, PPC) for specific customer segments, allowing businesses to allocate budget toward high-performing channels and optimize overall marketing spend.
e. Forecasting Customer Lifetime Value (CLV)
Predictive analytics can help businesses forecast customer lifetime value (CLV), which estimates the total revenue a customer will generate over the course of their relationship with a brand. By predicting CLV, businesses can focus on acquiring high-value customers, and prioritize retention efforts to maximize long-term profitability.
For example:
- E-commerce companies can use predictive analytics to determine which customers are likely to become loyal repeat buyers and invest more in customer retention strategies for those high-value customers.
f. Predicting Customer Churn and Retention
Churn prediction is one of the most valuable applications of predictive analytics. By analyzing historical data on customers who have unsubscribed, canceled services, or stopped purchasing, businesses can identify patterns that predict when a customer is likely to churn. Once at-risk customers are identified, businesses can intervene with targeted retention strategies such as discounts, personalized emails, or special offers.
For example:
- A subscription-based service can use predictive models to determine when a customer is likely to cancel their subscription and send them an offer to stay, such as a limited-time discount or a value-added service.
3. Techniques Used in Predictive Analytics for Marketing
Several key techniques and algorithms are commonly used in predictive analytics for marketing:
a. Regression Analysis
Regression analysis helps businesses understand the relationship between different variables and predict future outcomes. In marketing, it can be used to predict sales based on factors like advertising spend, seasonality, and customer behavior.
b. Classification Algorithms
Classification algorithms, such as decision trees or support vector machines (SVM), are used to categorize customers into different groups based on their likelihood to engage in certain behaviors, such as purchasing a product or unsubscribing from an email list.
c. Machine Learning and Artificial Intelligence (AI)
Machine learning and AI techniques, including neural networks, random forests, and deep learning, allow predictive models to continuously learn from new data and improve their accuracy over time. These algorithms can handle vast amounts of customer data and uncover complex patterns that might be overlooked by traditional methods.
d. Time Series Analysis
Time series analysis is used to predict future trends based on historical data points over time. Marketers can apply time series models to forecast sales, seasonal trends, website traffic, and more, allowing them to plan ahead and optimize marketing efforts for peak periods.
e. Natural Language Processing (NLP)
NLP techniques are used to analyze text data from sources like customer reviews, social media comments, and emails. This can provide insights into customer sentiment, preferences, and needs, which can be used for more personalized marketing campaigns.
4. Examples of Predictive Analytics in Action
Here are some examples of how predictive analytics is being used in marketing:
- E-commerce: Retailers use predictive analytics to recommend products based on customer browsing history, purchase frequency, and demographic data. Amazon’s “Customers who bought this also bought” feature is an example of predictive recommendations in action.
- Email Marketing: Email campaigns can be personalized using predictive analytics. Marketers can predict the best time to send emails based on past engagement patterns and send personalized offers or recommendations based on past purchases or browsing behavior.
- Ad Targeting: Facebook and Google Ads use predictive analytics to display ads to users based on their likelihood to engage with the ad. Advertisers can fine-tune their targeting to ensure their ads reach high-potential customers.
- Retail: Brick-and-mortar retailers use predictive analytics to forecast demand for specific products and optimize inventory management. They can predict which items are likely to be in demand during particular seasons or sales events and adjust their stock levels accordingly.
5. Challenges and Considerations in Using Predictive Analytics
While predictive analytics offers immense potential, there are several challenges that businesses should consider:
- Data Quality: Accurate predictions depend on high-quality, clean data. Poor data quality, incomplete datasets, or inaccurate customer information can lead to flawed predictions.
- Overfitting: In predictive models, overfitting occurs when the model is too complex and fits the training data too closely, leading to poor performance when applied to new, unseen data.
- Privacy Concerns: Using customer data to predict behavior raises privacy concerns. Businesses must ensure they are complying with data protection regulations (such as GDPR) and obtain consent for data collection and analysis.
- Implementation Costs: Implementing predictive analytics tools can be resource-intensive, requiring advanced tools, skilled personnel, and significant investment in technology.
6. Conclusion
Predictive analytics is revolutionizing how marketers understand and engage with their customers. By forecasting customer behavior, marketers can make data-driven decisions that improve targeting, personalize content, and optimize campaigns for higher engagement and conversions. From predicting customer lifetime value and churn to optimizing lead scoring and ad spend, predictive analytics empowers businesses to stay ahead of the competition and create more impactful marketing strategies. However, to unlock its full potential, companies must ensure data quality, respect customer privacy, and use the right analytical tools to generate accurate insights.
#dataanalytics #datascience #data #bigdata #machinelearning #dataanalysis #datavisualization #datascientist #analytics #artificialintelligence #python #ai #technology #database #dataanalyst #business #deeplearning #programming #statistics #tech #sql #businessintelligence #datamining #coding #powerbi #excel #innovation #digitalmarketing #software #pythonprogramming #digitaltransformation #iot #computerscience #datadriven #businessanalytics #datamanagement #dataviz #cybersecurity #marketing #bigdataanalytics #datasciencetraining #cloudcomputing #sqlserver #ml #datasciencejobs #cloud #microsoft #dataprotection #mysql #datasecurity #tableau #java #dataengineering #businessanalyst #datacenter #dataengineer #programmer #dataentry #automation #developer #BuddyInfotech #Adindia360 https://buddyinfotech.in/ https://adindia360.in/