AI Implementation in Business Operations
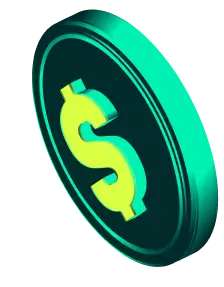
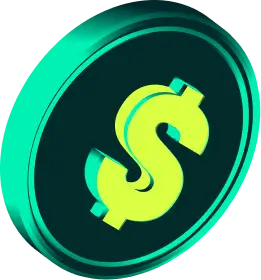
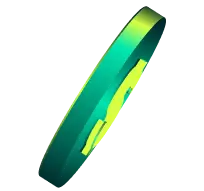
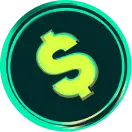
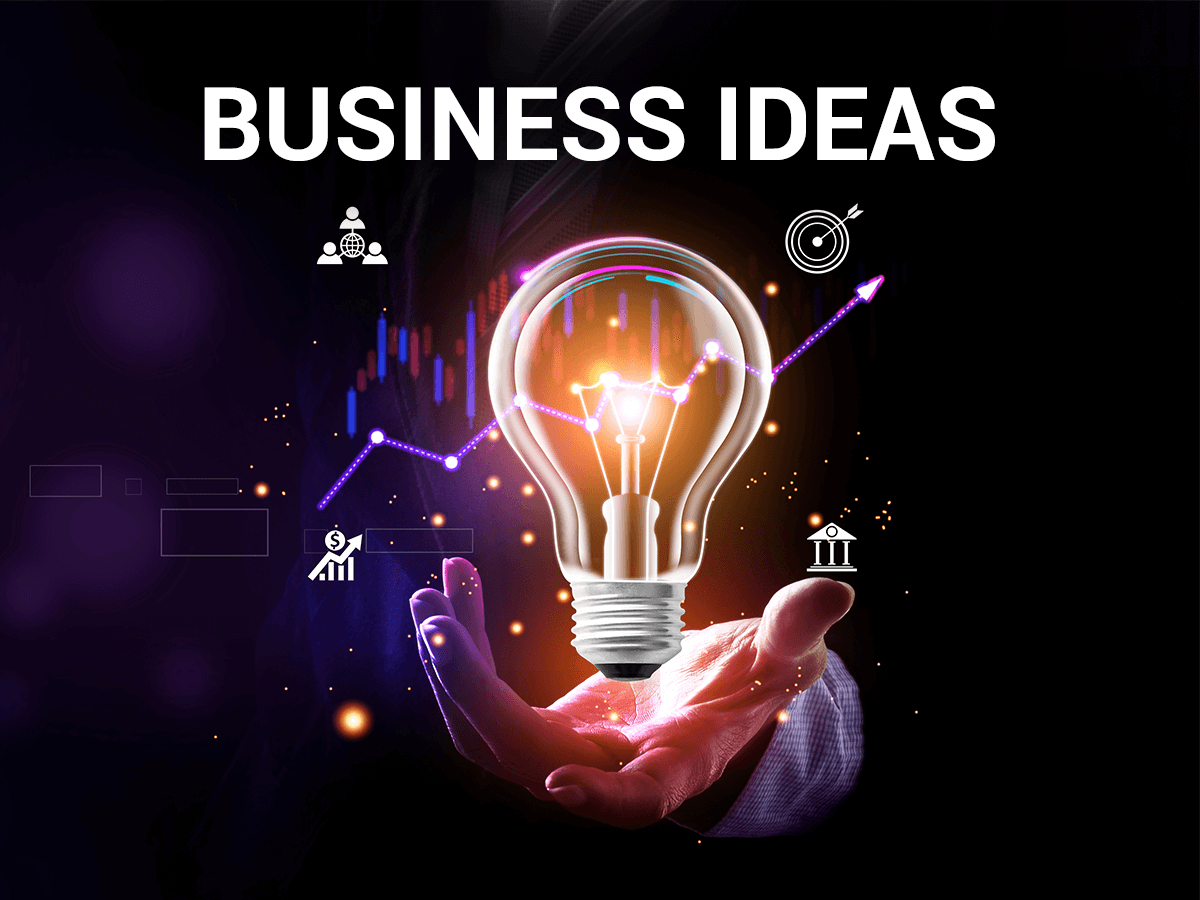
AI Implementation in Business Operations
AI Implementation in Business Operations
1. Introduction to AI in Business Operations
- Overview: Introduce AI’s growing role in transforming business operations across industries, focusing on productivity, cost savings, and strategic decision-making.
- Discussion Points:
- How AI integration goes beyond automation, impacting the entire value chain from production to customer service.
- Growing interest among businesses in AI-driven solutions for efficiency and competitive advantage.
- Objective of the Blog: To showcase AI’s practical impact on business operations through a real-world example in manufacturing.
2. Understanding AI’s Role in Operational Efficiency
- Overview: Explain how AI tools enhance productivity and streamline processes by automating tasks, analyzing data, and predicting outcomes.
- Key Benefits:
- Automation: Reducing human intervention in repetitive tasks, allowing employees to focus on high-value work.
- Data Analysis: AI-driven insights for predictive maintenance, demand forecasting, and inventory management.
- Process Optimization: How AI improves workflows, reduces downtime, and increases output quality.
- Actionable Insight: Examples of areas where AI can be applied in operations, such as logistics, quality control, and customer support.
3. Case Study: AI-Driven Transformation in Manufacturing
- Overview: Introduce the case study of a manufacturing firm that successfully integrated AI to optimize its production process.
- Background on the Firm:
- Briefly describe the company’s operational challenges (e.g., high production costs, inefficient workflows, or material wastage).
- Describe their goal of using AI to improve efficiency, reduce waste, and enhance production quality.
- Key AI Tools Used:
- Predictive Maintenance: AI systems that monitor machinery and predict failures before they occur.
- Quality Control Automation: AI-driven cameras or sensors that detect defects in real-time, reducing waste.
- Supply Chain Optimization: AI software for demand forecasting and inventory management to prevent overproduction or stockouts.
4. The Impact of AI on Productivity and Cost Reduction
- Overview: Discuss the measurable impact of AI implementation on the firm’s productivity and cost structure.
- Key Outcomes:
- Increased Throughput: Faster, optimized production cycles with fewer delays due to predictive maintenance.
- Reduced Waste: Real-time defect detection and inventory management cut down material waste significantly.
- Labor Efficiency: Automation allowed employees to shift from manual tasks to higher-skilled roles, enhancing overall productivity.
- Quantitative Results:
- Highlight any statistics or metrics from the case study, such as percentage reduction in waste, cost savings, or productivity improvements.
5. AI for Improved Decision-Making in Operations
- Overview: Explain how AI-powered analytics improved the company’s strategic decision-making process.
- Key Areas of Decision-Making:
- Real-Time Data Analysis: AI’s ability to provide actionable insights from vast amounts of data for more informed decisions.
- Demand Forecasting: Predictive analytics tools that anticipate customer demand, optimizing production and inventory.
- Risk Management: Using AI to identify operational risks (such as potential supply chain disruptions) before they impact production.
- Case Study Insights:
- How AI-driven analytics enabled the firm to make proactive decisions, reduce downtime, and improve planning accuracy.
6. Overcoming Challenges in AI Implementation
- Overview: Highlight the challenges faced during the AI integration process and how they were addressed.
- Key Challenges:
- Data Integration: Difficulty in gathering clean, structured data for AI models.
- Employee Adaptation: Resistance to change and need for training in AI-related tools and processes.
- Initial Costs: Investment in AI technology, tools, and infrastructure.
- Solutions and Lessons Learned:
- Training and upskilling employees to adapt to AI-driven workflows.
- Phased implementation to allow gradual adjustment and mitigate initial costs.
- Ensuring data quality and establishing clear goals to drive impactful AI outcomes.
7. Broader Applications of AI in Various Business Operations
- Overview: Expand beyond manufacturing to showcase other industries where AI is having an operational impact.
- Examples:
- Retail: AI for inventory management, customer insights, and personalized recommendations.
- Finance: AI in fraud detection, risk assessment, and automated customer service.
- Healthcare: AI-driven diagnostics, patient data analysis, and scheduling.
- Takeaway Insight: The versatility of AI applications across sectors and how AI tools can be adapted to meet diverse operational needs.
8. The Future of AI in Operational Efficiency
- Overview: Speculate on emerging trends in AI that are expected to shape future business operations.
- Key Trends:
- Edge AI and IoT Integration: AI-powered IoT devices for real-time monitoring and analytics in manufacturing.
- Hyper-Personalization: AI-enabled customization in production to meet specific customer preferences.
- AI-Driven Sustainability: How AI can support waste reduction, energy management, and sustainable practices.
- Final Thoughts: Encourage businesses to explore AI’s potential in their operations, highlighting the importance of staying agile and adaptable in a tech-driven world.
9. Conclusion and Key Takeaways
- Overview: Summarize the benefits of integrating AI into business operations, based on the case study and broader applications.
- Actionable Steps:
- Start with pilot projects to assess the impact of AI on specific operational areas.
- Focus on quality data and training to ensure successful AI implementation.
- Balance technology with human insight to maintain a holistic approach to decision-making.
- Encouragement: Urge readers to consider AI as a strategic partner that can significantly enhance business operations and productivity.
This outline provides a comprehensive guide on how AI can be implemented in business operations, using a manufacturing case study to illustrate real-world applications and results. Each section can be further developed with examples, statistics, or expert quotes for depth and credibility. Let me know if you’d like more detail on a specific aspect or additional case studies!